Google Cloud Is Reshaping AI Infrastructure to Meet the Demands of the Inference Era
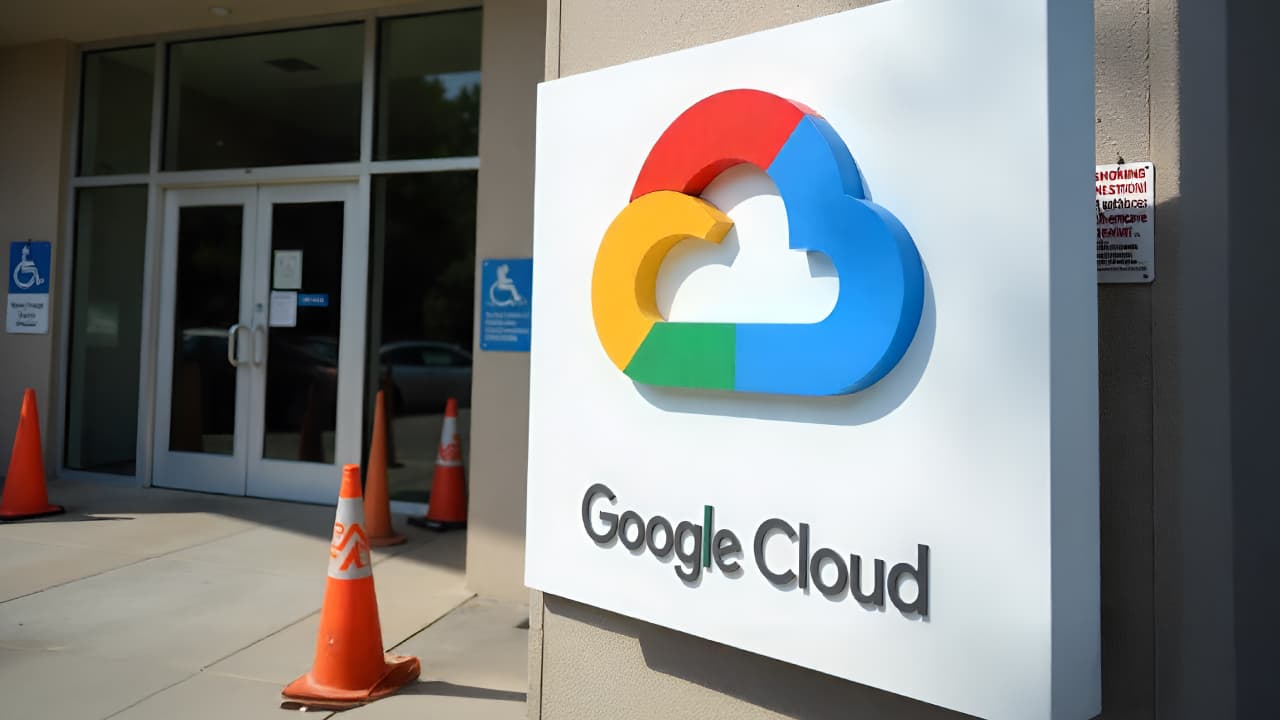
As artificial intelligence models grow increasingly complex, Google Cloud is reengineering its infrastructure to support a new generation of AI workloads. With rising demand from enterprises and the shift toward more reasoning-intensive models, the company is focused on improving cost efficiency and scalability.
2025 is positioned as a turning point. Google Cloud identifies it as the year of inference — a phase where AI models not only respond but reason across multiple steps. These models go beyond simple outputs, functioning more like agents embedded within larger, task-oriented workflows. As organizations integrate these capabilities, infrastructure requirements are shifting dramatically.
The Inference Era Demands New Performance Standards
Reasoning models require more computational power than traditional AI systems. They operate across complex decision chains and engage in agentic workflows that simulate multi-step human-like reasoning. Supporting such workloads necessitates infrastructure that is both powerful and adaptable.
Google Cloud is prioritizing inference performance, enabling enterprise customers to handle larger tasks at scale. A key development is the company’s focus on optimizing cost per inference — an essential factor for businesses operating AI-driven services. The goal is to reduce expenses while maintaining high performance across diverse tasks.
Also read: BAT VC Launches $100 Million Fund Targeting India’s AI and DeepTech Startups
One significant advancement comes through expanding support for VLLM (Versatile Large Language Model). Known for its speed and cost-efficiency on GPUs, VLLM is now being integrated with Google’s TPUs. This provides users with more flexibility, allowing them to switch between hardware accelerators depending on their workload and budget requirements.
Open Source Roots Power Enterprise AI
Google’s open-source legacy is playing a foundational role in its AI evolution. Projects like Kubernetes revolutionized software deployment, and now frameworks like JAX are powering modern AI training. Originally developed for internal use, JAX has been open-sourced to provide scalable, efficient support for training and serving large models, including Google’s own Gemini series.
As AI infrastructure continues to evolve, Google Cloud’s investment in flexible, open, and high-performance tools ensures it remains central to powering the next wave of intelligent systems.