Microsoft Unveils High-Efficiency BitNet Model for Lightweight AI Applications
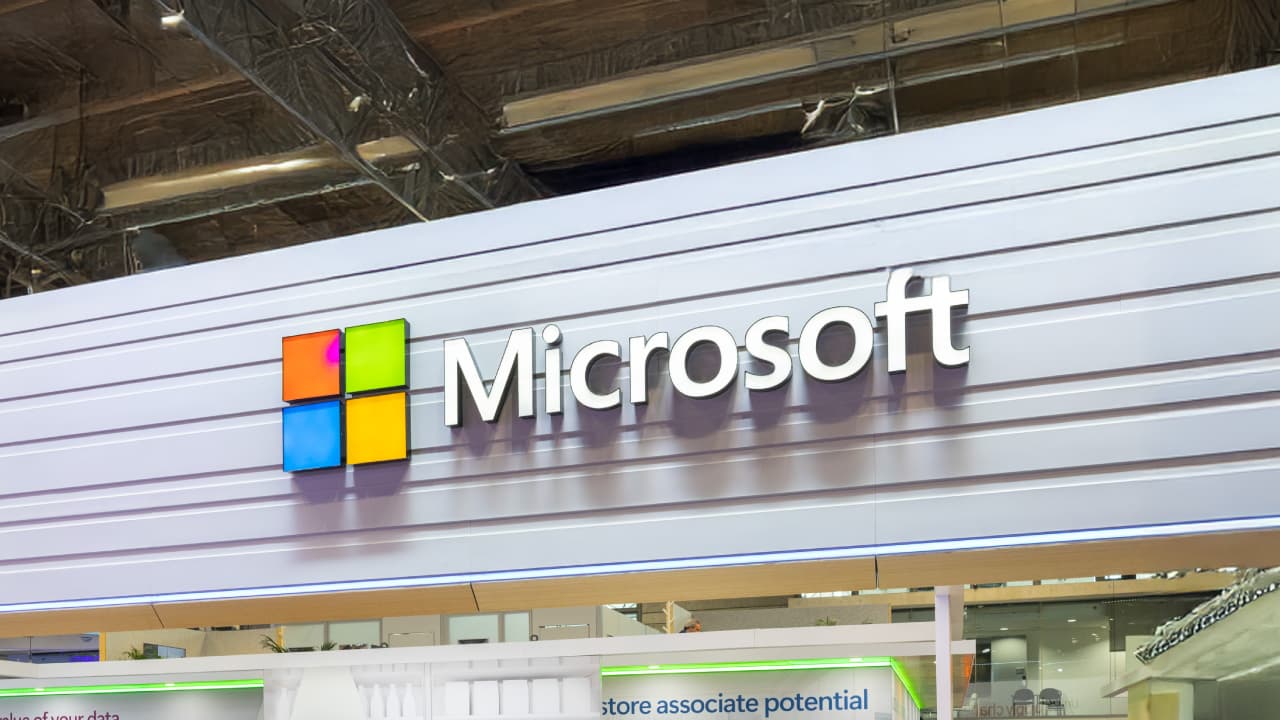
Microsoft researchers have announced a breakthrough in efficient AI modeling with the release of BitNet b1.58 2B4T, described as the largest-scale 1-bit AI model developed to date, as per TechCrunch. Released under the MIT open-source license, this bitnet model can run on CPUs, including Apple’s M2 chip, making it a notable advancement for devices with limited computing power.
What Are Bitnets?
Bitnets are highly compressed AI models that simplify computations using only three weight values: -1, 0, and 1. Unlike traditional AI models requiring more complex weight quantization, bitnets drastically reduce memory and computational demands, making them ideal for deployment on low-power or edge devices.
Model Details and Performance
According to TechCrunch, BitNet b1.58 2B4T, with 2 billion parameters, was trained on a vast 4 trillion-token dataset, equivalent to around 33 million books. Despite its compact structure, it matches or surpasses the performance of similarly sized models on key industry benchmarks, such as GSM8K (grade-school math) and PIQA (physical commonsense reasoning).
In head-to-head evaluations, Microsoft's BitNet surpassed Meta’s Llama 3.2 1B, Google’s Gemma 3 1B, and Alibaba’s Qwen 2.5 1.5B in performance. Furthermore, BitNet b1.58 2B4T demonstrated up to twice as fast inference speeds while consuming significantly less memory than conventional models of the same size.
The high performance of BitNet b1.58 2B4T depends on Microsoft’s custom software framework, bitnet.cpp, which currently supports only specific CPU hardware. Notably, GPUs, the standard for most AI infrastructure are not yet supported, limiting its use in mainstream training environments and large-scale production.
Also read: Google Rolls Out Veo 2-Powered Video Generation in Gemini
Outlook
BitNet b1.58 2B4T offers a compelling path forward for deploying efficient AI models on resource-constrained devices, especially where energy and memory efficiency are critical. However, hardware compatibility and ecosystem support remain key challenges that could slow broader adoption.