AI Model Identifies Predictors of TB in HIV Patients
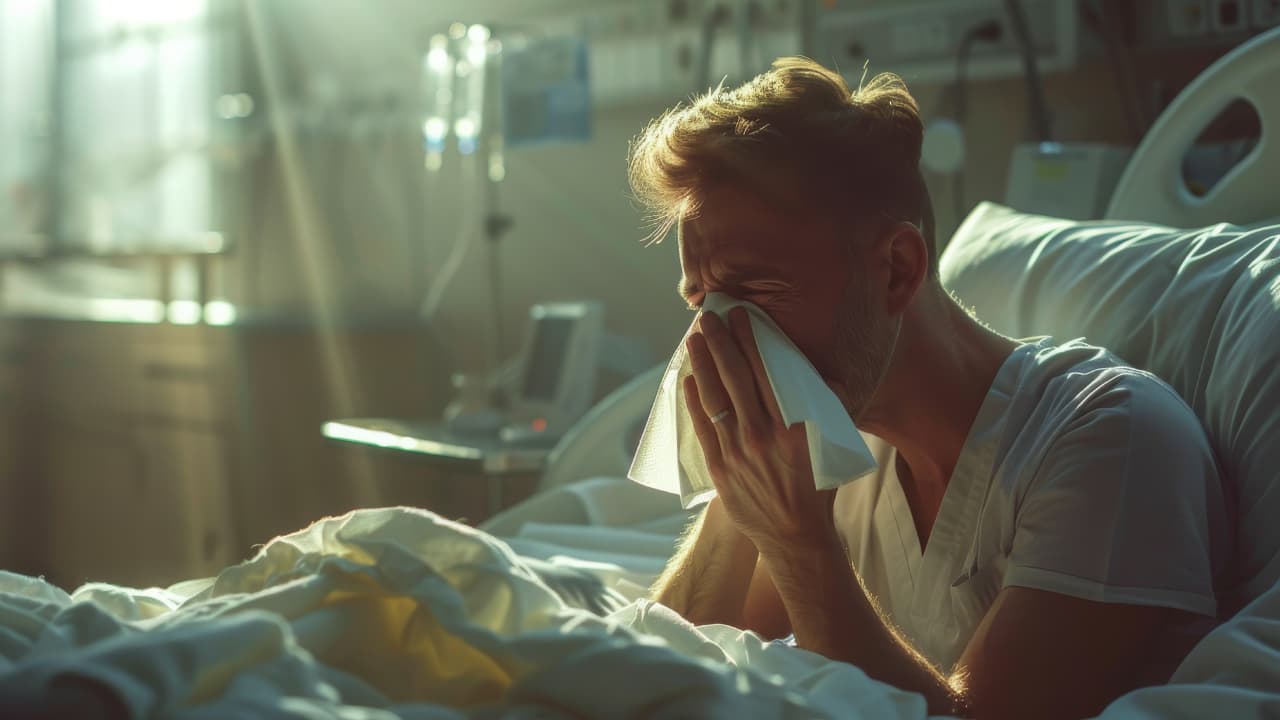
A Swiss artificial intelligence (AI) model has shown promising results in predicting which HIV-positive patients are at high risk of developing active tuberculosis (TB). The machine learning tool outperformed traditional biological tests and could offer a breakthrough in TB prevention strategies.
AI Outshines Traditional TB Testing
Current methods for identifying latent TB infections, especially in people living with HIV, often fall short. In Switzerland, tests like interferon gamma release assays (IGRAs) and tuberculin skin tests identify just 30% of individuals who later develop active TB. Dr. Johannes Nemeth of the University of Zürich described this performance as “worse than tossing a coin.”
That shortfall prompted Nemeth and his team to explore AI-based alternatives. Using data from the Swiss HIV Cohort Study, which covers around 70% of HIV patients in the country, they trained a random forest machine learning model. The model analyzed over 23 years of clinical data, including immune function, biomarkers, and demographic details.
Also read: Alliance for Healthcare from the Eye Launches with a Vision to Revolutionize Healthcare through AI and Ocular Data
Predicting TB With Greater Accuracy
Unlike conventional tests that depend heavily on immune response—often impaired in people with HIV—the AI model reviewed a broader range of health indicators. These included metabolic markers like glucose, cholesterol, and creatinine, as well as body mass index and mean arterial pressure.
The initial model, using 48 variables, achieved 70.1% sensitivity and 81.0% specificity. A simplified version using 20 variables still performed well, with 57.1% sensitivity and 77.8% specificity—outclassing the sensitivity of biologic tests by a wide margin.
This performance, combined with its use of routinely collected data, makes the AI model a cost-effective, scalable alternative to existing TB screening methods.
One Size Doesn’t Fit All
When the model was tested on a similar cohort in Austria, initial results were disappointing. Researchers found that migration patterns affected the outcome: TB patients in Switzerland were mostly from sub-Saharan Africa, while in Austria they were primarily from former Soviet states. Adjusting variables for ethnicity and region of birth improved model performance, emphasizing the need for local calibration.
Dr. Emily Wong, a TB and AI expert not involved in the study, praised the findings. “This opens one’s eyes to the idea that large datasets with clinical variables can predict TB risk meaningfully,” she noted.
What’s Next?
Dr. Nemeth is planning an implementation study to test whether the model’s risk scores will influence physicians to act—offering TB tests or preventative treatments like isoniazid or rifampicin.
Wong added a note of caution: decisions around TB prevention must balance potential benefits and risks, including side effects. However, she agrees that AI-driven tools could eventually support clinician decision-making in real-time.